Flinders University: AI Guides Evolution of Adaptive Control Systems
Unmanned Underwater Vehicles (UUVs) are used around the world to conduct difficult environmental, remote, oceanic, defence and rescue missions in often unpredictable and harsh conditions.
A new study led by Flinders University and French researchers has now used a novel bio-inspired computing artificial intelligence solution to improve the potential of UUVs and other adaptive control systems to operate more reliability in rough seas and other unpredictable conditions.
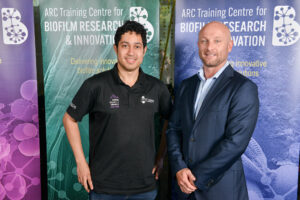
This innovative approach, using the Biologically-Inspired Experience Replay (BIER) method, has been published by the Institute of Electrical and Electronics Engineers journal IEEE Access.
Unlike conventional methods, BIER aims to overcome data inefficiency and performance degradation by leveraging incomplete but valuable recent experiences, explains first author Dr Thomas Chaffre.
“The outcomes of the study demonstrated that BIER surpassed standard Experience Replay methods, achieving optimal performance twice as fast as the latter in the assumed UUV domain.
“The method showed exceptional adaptability and efficiency, exhibiting its capability to stabilize the UUV in varied and challenging conditions.”
The method incorporates two memory buffers, one focusing on recent state-action pairs and the other emphasising positive rewards.
To test the effectiveness of the proposed method, researchers conducted simulated scenarios using a robot operating system (ROS)-based UUV simulator and gradually increasing scenarios’ complexity.
These scenarios varied in target velocity values and the intensity of current disturbances.
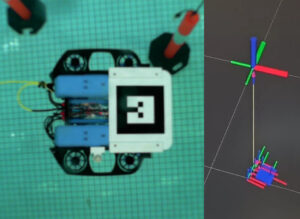
Senior author Flinders University Associate Professor in AI and Robotics Paulo Santos says the BIER method’s success holds promise for enhancing adaptability and performance in various fields requiring dynamic, adaptive control systems.
UUVs’ capabilities in mapping, imaging and sensor controls are rapidly improving, including with Deep Reinforcement Learning (DRL), which is rapidly advancing the adaptive control responses to underwater disturbances UUVs can encounter.
However, the efficiency of these methods gets challenged when faced with unforeseen variations in real-world applications.
The complex dynamics of the underwater environment limit the observability of UUV manoeuvring tasks, making it difficult for existing DRL methods to perform optimally.
The introduction of BIER marks a significant step forward in enhancing the effectiveness of deep reinforcement learning method in general.
Its ability to efficiently navigate uncertain and dynamic environments signifies a promising advancement in the area of adaptive control systems, researchers conclude.
Learning Adaptive Control of a UUV using A Bio-Inspired Experience Replay Mechanism (2023) by Thomas Chaffre, Paulo E Santos, Gilles Le Chenadec, Estelle Chauveau, Karl Sammut and Benoit Clement has been published in IEEE Access, an Institute of Electrical and Electronics Engineers journal DOI: 10.1109/ACCESS.2023.3329136
Acknowledgements: This work was funded by Flinders University and ENSTA Bretagne with support from the Government of South Australia (Australia), the Région Bretagne (France) and Naval Group.