ITMO Develops New AI-Based Method for Converting Medicine into Pills
ITMO researchers have developed a machine learning-based algorithm that will make the production of finished dosage forms easier and cheaper. Thanks to the new solution, even before experimental tests it will be possible to generate accessory molecules with the necessary properties and assemble them into a structure of a treatment – a pharmaceutical co-crystal. The results of the research behind the algorithm will be presented for the first time at a dedicated workshop of the prestigious AAAI Conference.
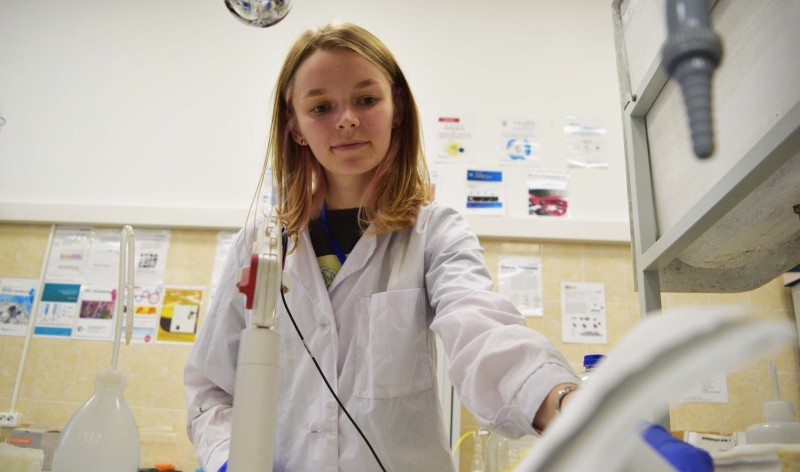
AAAI Conference is taking place in Canada on February 20-27. The event is focusing on developing theoretical and applied research in AI and is meant to foster experience exchange between researchers and practicians. Supported by the American Association for Artificial Intelligence, AAAI Conference ranks at the top of ShanghaiRanking’s Academic Excellence Survey and is also featured in the CORE ranking as an A* conference (the most prestigious rank).
As AI is a rapidly developing field, participation in conferences like AAAI equals a publication in a high-ranking journal. One of the projects that ITMO University will present at the AI2ASE workshop of AAAI is called Generative AI for Co-Crystal Design with Property Control.
Developed by researchers from the university’s ChemBio Cluster (Center for AI in Chemistry) and the Laboratory of Computational Modelling of Natural Systems (NSS Lab), the new algorithm is capable of generating co-crystals – crystals made from several molecules. With the new solution, it will be possible to cut down on the number of experiments, thus making the production of treatments faster and cheaper. For instance, the algorithm will help improve the tabletability of medicines, which is the process of compressing a pharmaceutical substance into a tablet.
“Pharmaceutical co-crystals contain a drug molecule and a coformer, an assistant molecule that helps change the physical and chemical properties of the pharmaceutical. Usually, it takes a great number of experiments to select a coformer, which means that developing co-crystals with the necessary properties is a lengthy process. Our algorithm for automated search of co-crystals in silico can help significantly accelerate it,” shares Nina Gubina, one of the project’s authors and an engineer at ChemBio Cluster.

Nina Gubina. Photo courtesy of the subject
First, the algorithm determines co-crystallization candidates and evaluates their mechanical properties; then it selects the best molecules and improves them with an evolutionary algorithm. Currently, the project is written in Python and can be used as a basis of a website or an app that will make co-crystal development easier, quicker, and cheaper. In the future, the team wants to experimentally test the algorithm, train it to predict more co-crystal properties, as well as adapt it for work with other chemical systems.
“In our work, we relied on a hybrid method: we applied a generative neural network and fine-tuned a crystal’s structure using evolutionary optimization. Thanks to this, we were able to capitalize on the advantages of generative AI and intelligent optimization methods, ensuring the high quality, variability, and originality of the resulting crystal structures. At the same time, we applied the GOLEM open library developed at ITMO’s Research Center “Strong AI in Industry,” says Nikolay Nikitin, one of the project’s authors, a researcher at NSS Lab, and the head of the frontier laboratory Automated Machine Learning.
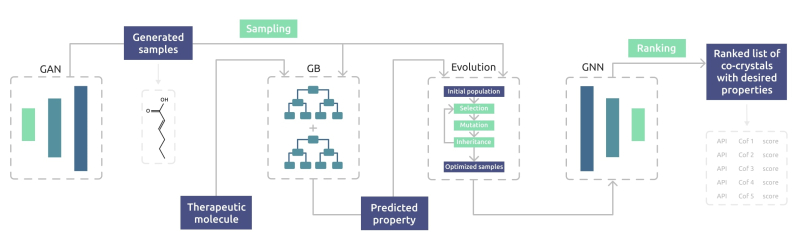
The scheme of the algorithm. Credit: ai-2-ase.github.io
A total of five research articles from ITMO have been accepted for the conference’s workshops this year. Among them are ones focused on predicting sea ice dynamics in the Arctic, automated design of graph-based models and structures using evolutionary modular approach, generation of topological properties in automated machine learning for time series prediction, and development of synthetic graphs using evolutionary optimization.
Project authors: Nina Gubina, Andrei Dmitrenko, Ivan Lebedev, Nikita Serov, Vladimir Vinogradov, Lyubov Yamshchikova, Grigory Kirgizov, Nikolay Nikitin.