Unleashing Potential: Exploring the Opportunities of AI in Revolutionizing Food and Ecosystems
Wageningen research groups are working with artificial intelligence (AI) more and more. What opportunities and dilemmas does that present for our research? And how can WUR invest in so-called ‘responsible AI’?
Wageningen research groups are producing and using more and more data. These data are fundamental to our understanding of food systems, ecosystems, and other processes studied at Wageningen. AI provides many new ways of interpreting these data.
For example, the Netherlands Plant Eco-phenotyping Centre (NPEC) in Wageningen takes enormous amounts of plant measurements under controlled conditions. By growing and measuring multiple crop varieties under different environmental conditions, they try to find out how the interaction between DNA and the environment works. These measurements generate an enormous amount of data, already more than 1,000 terabytes.
NPEC is currently using AI, according to Professor Mark Aarts and Rick van de Zedde of NPEC. Plant researchers take images of the plants in the greenhouse. An AI programme provides a filter on these images so that only the relevant plant parts are analysed. Irrelevant aspects like the background, pots and sticks are filtered out. But now they are discovering that they can extract more information from their amounts of data.
Plant disease detection
In another project, researchers are working on plant disease detection. The researchers grow different varieties in the greenhouse, introduce a pathogen and then follow the health condition of the plants. They use a range of fully automated imaging systems to determine whether the plants are sick and, if so, how sick they are. Now, they are training an AI system to recognise the disease stages on camera images since, using AI, computers can detect diseases earlier and more accurately than we can with the naked eye.
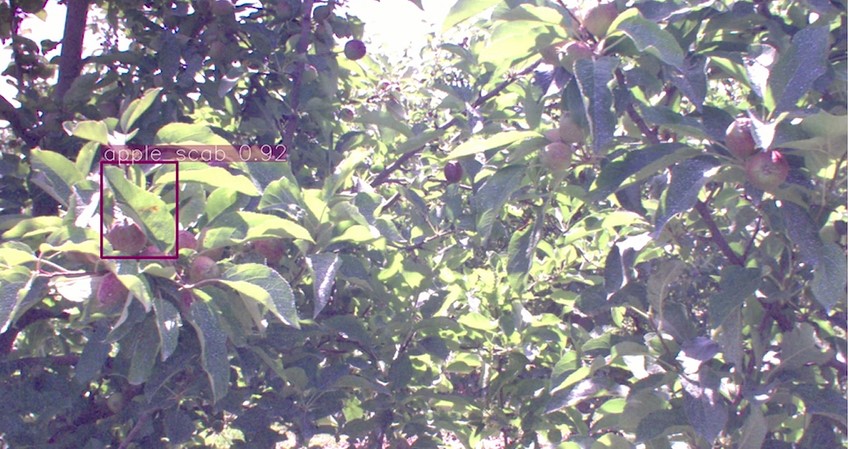
AI can also help find and explain strange deviations in tests. If the data for some plants deviate from the average, is this because they have received less water (the tube was clogged) or because that genotype responds differently to the treatment? Researchers often only see such deviations at a later stage, and then the meaning can no longer be determined, especially in large data sets. With AI, the computer can immediately identify and analyse this or search it in the database.
WUR appointed three personal professors in the field of artificial intelligence (AI) and data science over two years ago. Anna Fensel, Ricardo da Silva Torres, and Ioannis Athanasiadis work in various chair groups with support from the Wageningen Data Competence Center. They create, develop and apply AI solutions for issues in the Wageningen domain.
Professor Da Silva Torres has been involved in some of the seven Data Science and AI fellowship projects in recent years that aimed to strengthen AI in Wageningen research fields. Da Silva Torres collaborated with the Aquatic Ecology and Water Quality Management group on one of the projects. This group researches the resilience of ecosystems and possible tipping points if that resilience decreases.
The resilience of a coral reef
The group wanted to use remote sensing images and determine the resilience of an ecosystem using Turing patterns. A Turing pattern is a mathematical explanation for the emergence of patterns in biology from the interaction of two variables. Using such patterns, the researchers wanted to determine, for example, the resilience of a coral reef and whether that ecosystem was about to die. Da Silva Torres contributed AI knowledge; he was involved in the development of algorithms that assess and classify remote sensing images in terms of resilience.
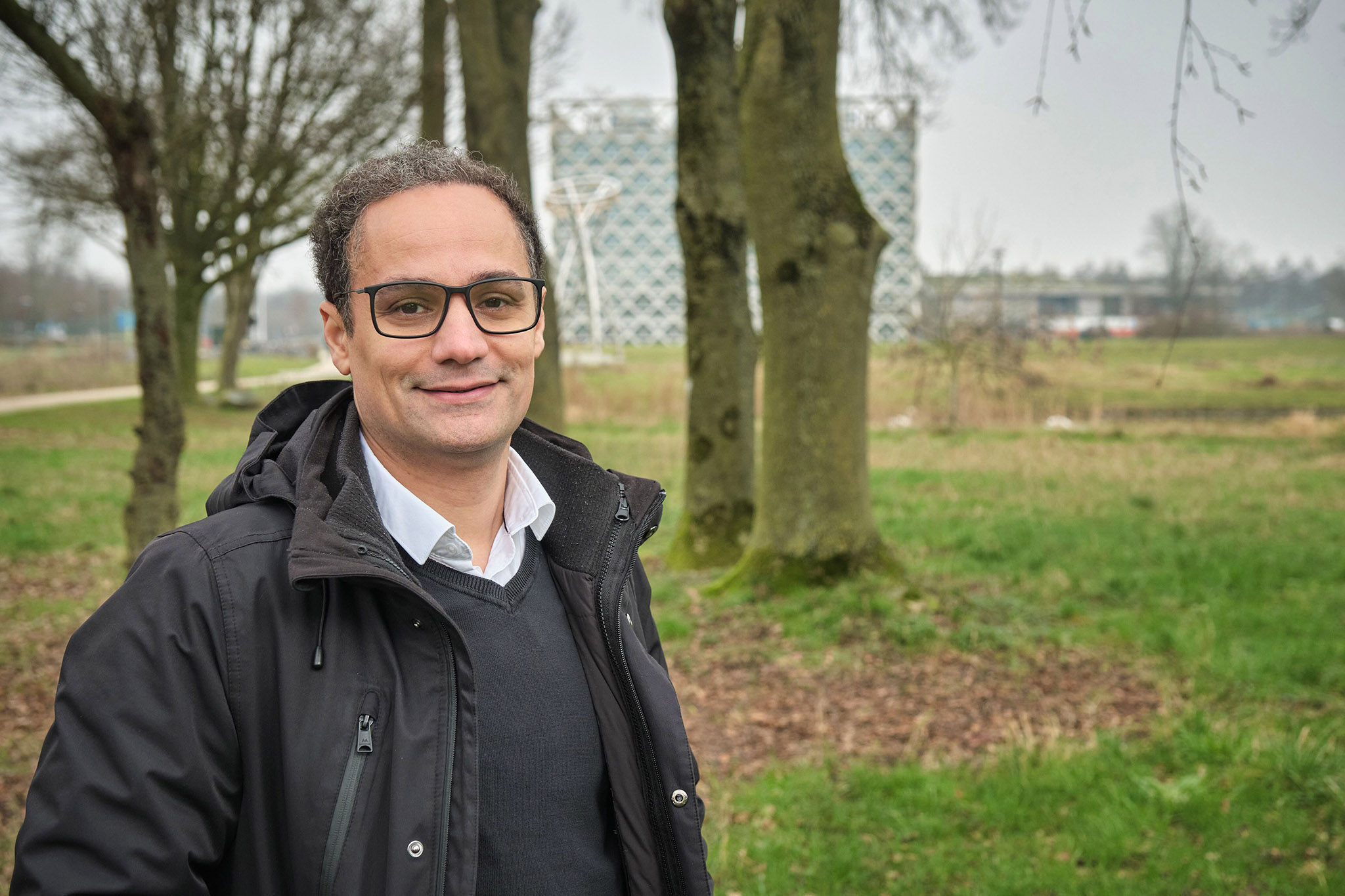
With such an algorithm, you can also determine the resilience of a savannah or rainforest based on satellite images, says Da Silva Torres. For his research, the domain knowledge of chair groups is crucial to creating a good algorithm. The research groups can provide the correct context and variables and indicate which properties of an entity are relevant. Moreover, they can test the algorithm using their research expertise. This collaboration between domain and AI experts benefits both and provides many opportunities in both directions.
The interaction between humans and machines is crucial, according to the AI professors, because many AI developments cannot be fully automated due to the risk that the computer will understand and classify data incorrectly. To keep track of the development of AI, it is therefore important that the origin and use of data are transparent. In other words, FAIR: findable, accessible, interoperable and reusable. This makes FAIR a sustainable alternative to some of big tech companies’ current data management practices.
Test centres for algorithms
Secondly, it is important that we transparently record the instructions and algorithms. EU legislation now requires Member States to categorise the algorithms and indicate how dangerous they are. The EU is also financing test centres that will test algorithms. WUR is part of a European facility for testing and experimenting with AI applications in the agri-food sector. Professor Ioannis Athanasiadis and colleagues from Agricultural Biosystems Engineering Group, Laboratory of Geo-Information Science and Remote sensing, and Wageningen Research are involved in this test facility.
Thirdly, we need to answer a question: who owns the data and which AI do we actually want? This involves many ethical, legal, and societal aspects (ELSA) of AI, which are also extensively researched at WUR. Wageningen technology philosopher professor Vincent Blok and Professor Da Silva Torres are involved in this. ELSA is a virtual lab, Blok emphasises; it experiments with the ethical, legal and social aspects of AI in concrete practices.
For example, Blok is researching milking robots. The farmers use them for milking, but the milking robots can contain AI and can, therefore, also be used for animal health and medical diagnostics. Do the farmers want that? Let’s say there is an algorithm that assesses the animal health. Who owns that data, and who is responsible if a cow remains ill or even dies? The ELSA lab raises these questions to include in the design process of a smart AI milking robot.
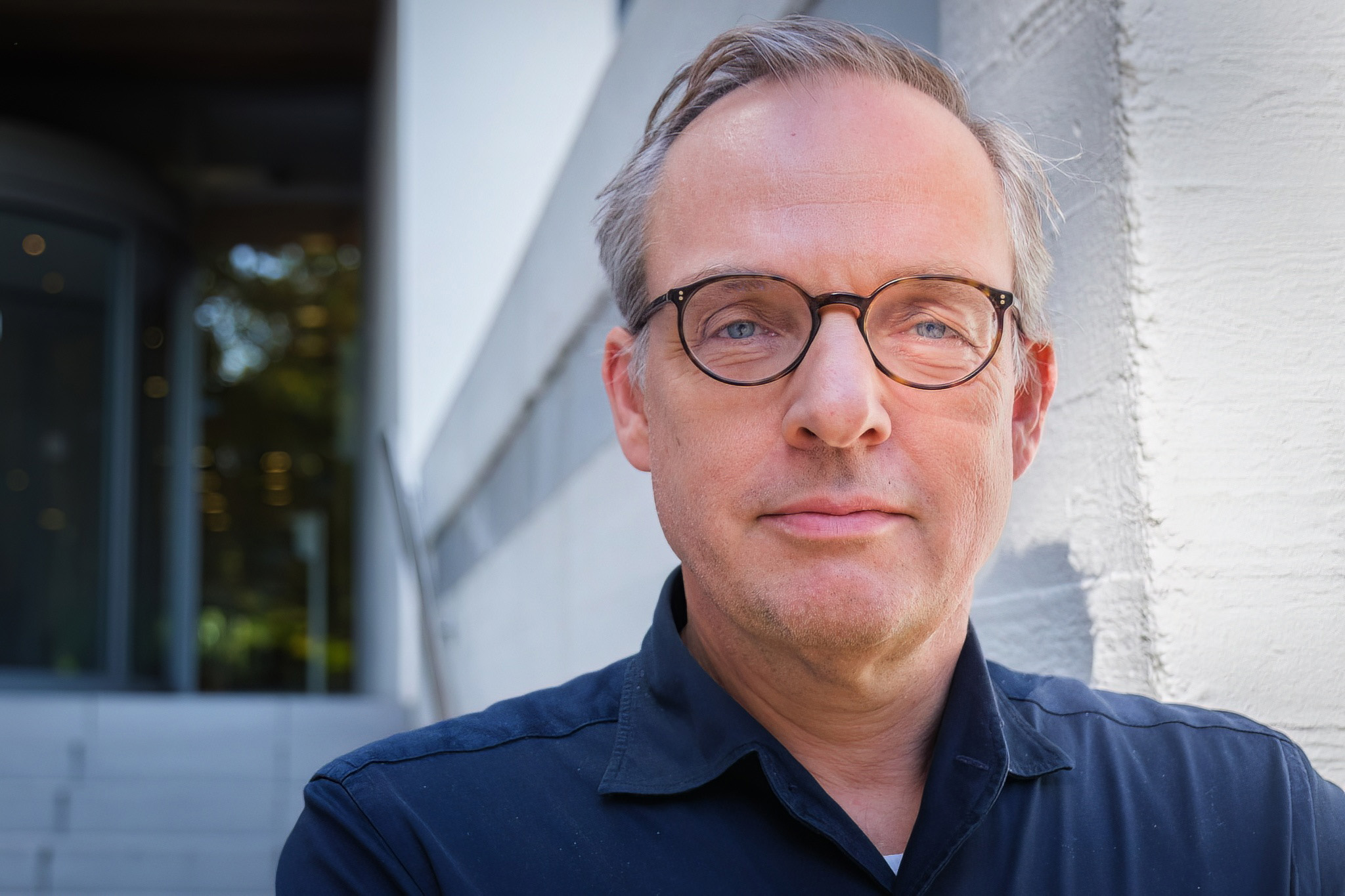
One issue in this design process is that AI is a decision support system – it gives the farmer advice. But does the farmer believe this advice? To what degree can they rely on such advice? Blok: ‘For that reason, you have to ensure that AI is not a black box; you have to be able to clearly explain how AI arrives at the advice. You may also need to be able to bring in the farmer’s expertise into the AI system and take it into account.’ In this way, AI becomes an interface between technology and behaviour.
There are more examples of this so-called ‘responsible AI’. AI and data science professor Anna Fensel has been creating and developing technical solutions for responsible data access and use. Such solutions have included ontologies and tools for data sharing compliant with the legal bases of the General Data Protection Regulation of the EU and further conditions (consent, contract, license-based data sharing). Suppose you drive a car. As a motorist, do you want to share data about your journey for road safety? Probably. This way, you achieve ‘positive data sharing’. Consumers can also share data for a sustainable future, or patients can share data about diseases, lifestyles and behaviours in a FAIR and transparent manner for purposes that would benefit everyone. In each case, however, it is essential to consider what data to share, how, with whom, and for how long.
Facial recognition
Challenges also arise from the huge influence of a few very large companies on technology development. The ELSA process alone cannot solve such challenges. For these, we should look at government involvement, preferably international. The EU AI Act, for example, regulates the power and competition of big tech companies and, as an example, requires consumer consent when using AI for facial recognition.
According to Blok, when considering research on facial recognition, it is not about a ban but about responsible use. It could be useful in nutritional research, in particular. A lot of this research to investigate what people eat is now done with questionnaires, which we know people do not complete correctly. Besides, the researchers cannot do classic experiments with consumers where, for example, one group eats a lot of sugar and fat, and the other doesn’t. This type of research is not allowed because the assumption is that eating a lot of fat and sugar is unhealthy. A smartwatch that tracks the consumption of test subjects could offer a solution.
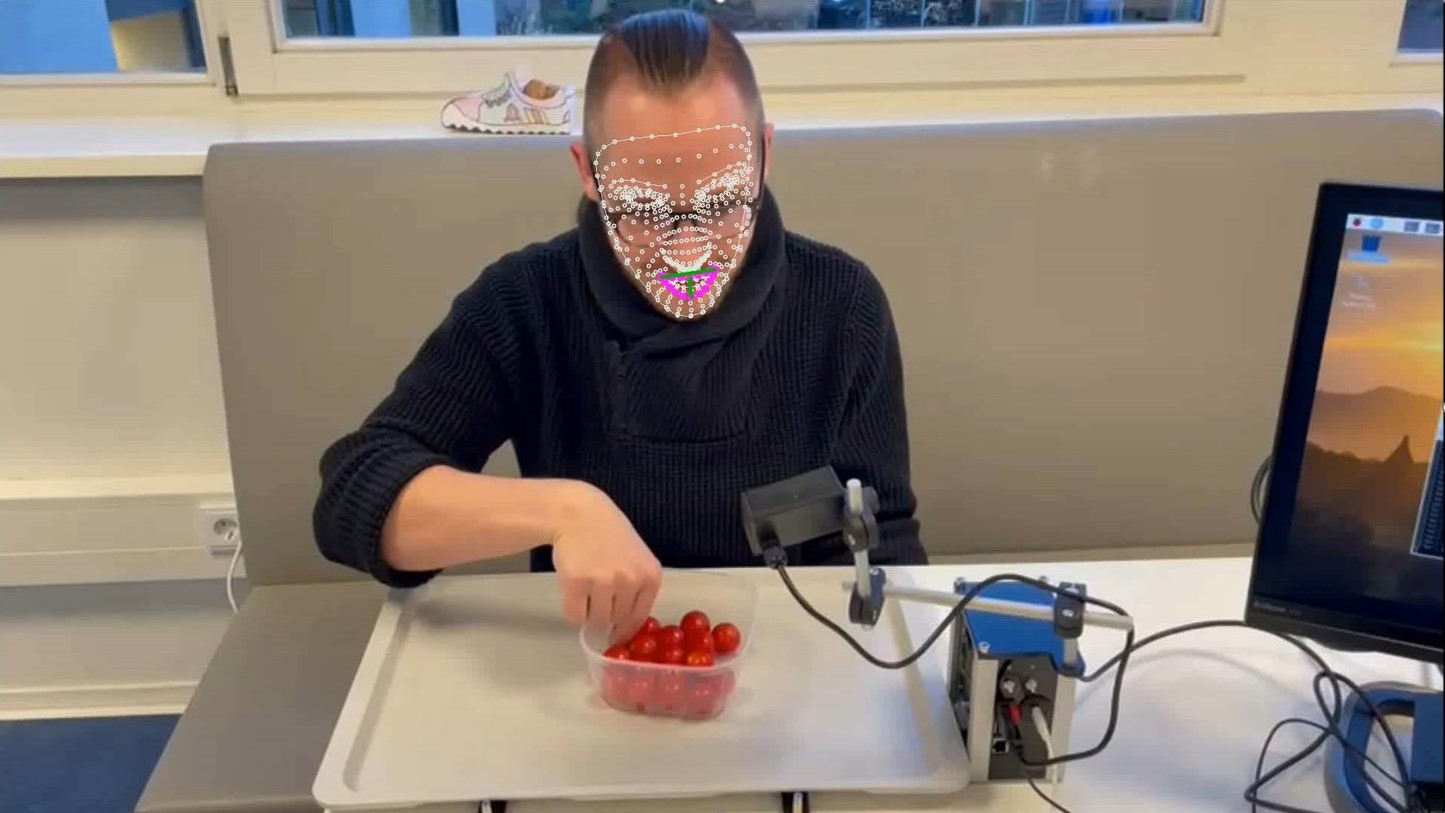
One step further is that nutrition researchers install cameras in nursing homes to see how much and what residents eat and how long they chew the food, says Blok. This is not possible without the consent of the residents. An option to deal with this is to have the cameras only register the food intake and chewing movement of a part of the face around the mouth. So, in this case, you can create AI protocols and interfaces that limit the camera images so that nutritional research is possible without facial recognition.
How are these AI protocols and interfaces actually created? That is the research area of AI professor Anna Fensel. She works on knowledge graphs. Knowledge graphs organise and connect information so that researchers can make meaningful connections and discover valuable insights. This creates an extensive knowledge network and serves as a foundation for FAIR data, delivering numerous benefits. For example, for researchers, knowledge graphs can be an alternative to manually sifting through large amounts of literature to find relevant information and better understand the bigger picture. An example of such a knowledge graph approach is currently being developed at WUR in the Horizon Europe SoilWise project in order to enable the aggregation of soil health data and information.
These knowledge graphs are semantic networks, Fensel says, using the meaning of words and symbols to structure data, complying with the web, semantic web and linked data formats and principles. This type of AI is essentially not about zeros and ones but about language, identity, and meaning. Semantic networks ensure that information gains meaning through the collaboration between humans and machines.
Digital tomato
Philosopher Vincent Blok also applies this approach to the tomato. Blok: ‘We are doing a project with digital twins, digital copies or representations of real things, to experiment with these copies. As an example, we have made a digital copy of a tomato. But what data do you need for that? The plant and food scientist will say: we need information about the shape, colour, water content and vitamins. The supermarket mentions uniformity and price, and the consumers mention different criteria. You soon discover that there are many implicit definitions and characteristics to capture a digital tomato – it is not a neutral representation, although engineers often think so. Decisions about the desired tomato are often made for commercial reasons, and defining the digital tomato makes those reasons visible.’
But in the next phase of AI, the computer may propose new tomato varieties with limited instructions from humans, using hypotheses from collected observation data. At NPEC, this future is already within reach. Mark Aarts: ‘The next step is to recognise patterns from large data files that the researchers had not yet noticed, if only because of the sheer quantity of data to be interpreted. Then, we can research AI-generated hypotheses. For instance, AI identifies a pattern and asks researchers: is this an interesting pattern? We are convinced that this will play an important role in plant breeding in the future.’
Is there still work for the plant researcher in the next phase? Aarts: ‘Yes, the domain experts remain crucial in the design and application of AI methods for data interpretation together with AI experts so that we can advance domain knowledge as well as AI methods.’